In memory of Bill Slawski.
This SEO Case Study has been written with 27 articles, by taking traffic from Healthline.com, Webmd.com, and Medicalnewstoday.com in 30 days. The early results are below.
- 20,000 New Queries in 30 days.
- 3,000 New Queries on the first web page.
- 13,000 New Queries between 10-50 rankings.
- 847% Organic Impression Increase
- 142% Organic Click Increase
The latter results are even better, but we will focus on the first 30 days with 27 articles. The rest of the article might be more academic for search engine understanding. But since this is an SEO Case Study section from my future Semantic SEO Course, it will have a lighter explanation.
To know when the Semantic SEO Course is out, subscribe to the Holistic SEO newsletter: https://www.getrevue.co/profile/koraygubur

Koray Tuğberk GÜBÜR is the CEO of Holistic SEO, a search engine optimization agency that focuses on understanding search engines, holistically.
What are quality thresholds and predictive ranking?
Quality thresholds and predictive ranking based on Information Retrieval are relevant to each other to determine a source’s overall ranking potential in an inverted index. The quality threshold involves a score for being ranked for a certain query, or network of queries, while predictive ranking helps quality thresholds to change, and improve, with continuous testing of the results.
Predictive ranking is called Predictive Information Retrieval by certain sources, such as Google. In other academic papers, the term “Uncertain Inference” is used. Even if they are not exactly the same, they are extremely similar.
In this article, a sample SEO Case Study will be shown from the E-A-T and Topical Authority point of view to demonstrate quality thresholds and predictive ranking.
Background of the SEO Case Study
The SEO Case Study to explain the quality thresholds of the search engines is an e-commerce website that sells luxury products that are related to health. The product is related directly to health.
The purpose of the project is to increase the organic traffic via semantic SEO to improve the conversions and make investments that will grow the brand bigger.
Below, you will see the SEMrush result graphic.
Below, the Ahrefs result graphic can be seen.
Below, you can see the 1 month later version of the subject website from Ahrefs.
The GSC data for the sample website is below. Despite the query count increase, the average position didn’t decrease, it continued to increase.
To see the overall background of the Quality Threshold SEO Case Study, you can watch the video below.
Why does a search engine need a quality threshold?
Quality thresholds are used in nearly all ranking, filtering, and sorting algorithms based on certain metrics.
For example, to classify a 800×600 image, a machine learning algorithm needs 58 million different “bundle boxes”, but it is not possible to do it because it is costly. Thus, search engines use “Region Proposals” with “Selective Search” for object detection. And, if there are 5 different objects, it will be hard to label the image. Thus, a threshold is a must.
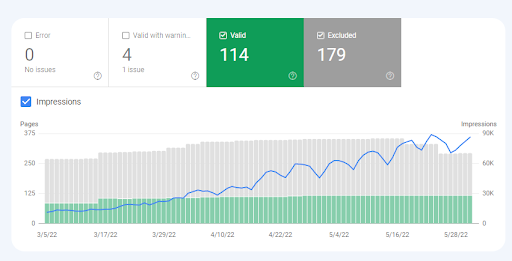
This is a small website
Another example of quality thresholds are supplemental indexes. The Charles Darwin of SEO, Bill Slawski, was the first person who mentioned the concept of the supplemental index by Google. According to design, Google uses a supplemental index and the main index. If a source exceeds the quality threshold, it changes its position from the supplemental index to the main index.
Let’s look at another example of the quality threshold that happens with indexation. Google uses predictive quality understanding by checking the initial URLs from the crawl queue to see whether it’s worth a deeper crawl or not.
A final example of the quality threshold is from News SEO; if Google doesn’t see a proper summary at the introduction of the article, they can skip the article for further ranking purposes. Thus, the quality of the summary can affect the overall ranking, even if it is not the quality of the entire article.
Note: The actual version of the SEO Case Study will be given during the Semantic SEO Course with 5 more websites, actual research papers, and more information.
Some other sample websites and industries for initial ranking with exceeded quality thresholds
Before moving further, you can see some other website samples that exceed the quality thresholds from different industries.
The sample below is an e-commerce store for certain gift products, and there is a planned launch. Thus, it has been indexed and served faster.
The example below is again from a YMYL website with a quick launch for exceeding quality thresholds.
The sample below is from a health industry website. You can see that “predictive ranking” works with different confidence levels.
The sample below is another quality threshold exceeded, and it is a local search directory for offline businesses for commercial activities. Its main benefit is stocking information.
The last sample below is an exact match domain for YMYL Industry, used cars.
These samples will be given in the Semantic SEO Course with more detailed scientific explanations. All these launches focused on specific types of query networks with an understanding of the ranking algorithms of Google, and quality thresholds with predictive ranking. Since all the samples are YMYL websites and touch the health, it is more crucial to state that it is possible to take traffic from global authorities without waiting that long.
Why is index partition necessary for a search engine?
Index partition means that a search engine divides its index into multiple parts. When a user enters a query to a search engine, do you think that a search engine checks the entire index? Then, you would wait two hours for a single SERP. And, what if a search engine searches the possible queries from the users even before the user searches for it to create document clusters? This is called synthetic queries, which I explained in my Semantic Search Engine and Query Processing methodology. The search engine’s purpose with index partition is to decide which document and query will go to which cluster of concepts. And, there are different index partition methods. The latest methods focus on Triples (Subject, Predicate, Object). Previous samples focused on the context terms and head words in the documents.
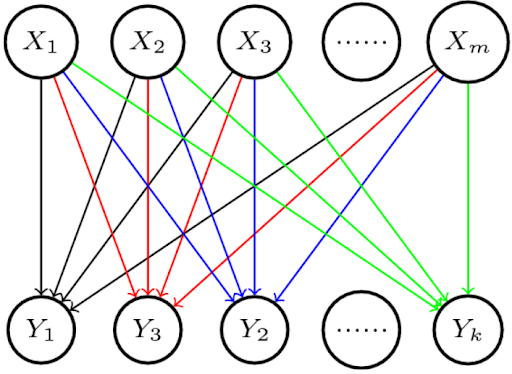
An example of Uncertain Inference for queries
Index partition and quality thresholds are related to each other.
How to decide which document goes to which cluster?
A search engine can index a document, then de-index and re-index it for another query. A query in the form of a verb can have different lemmatizations, and the search engine can create different index partitions for different verb formats. The document might not have the specific verb formats, or the specific verb + noun sequences, or even if it does, it might give incorrect information which contains the topic of a knowledge base. Thus, different quality thresholds are determined for different formats of the nouns, verbs, and other types of words such as adjectives.
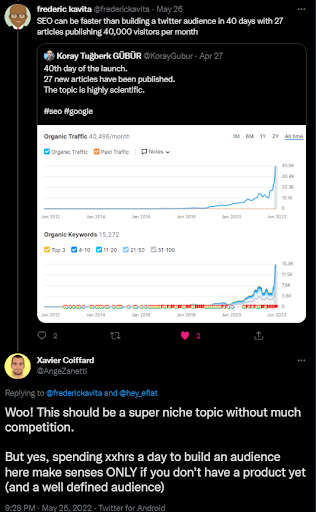
I didn’t write this case study against these claims. But I want to change how SEO is being done.
Relevance works to decide the overall connectedness between a document and the query. If the document has multiple macro and micro-contexts, it might be a contextless, or wrongly formatted document. For these types of situations, the search engine has passage indexing, which is a method to eliminate most of the irrelevant sections of the document and rank it with only that specific passage.
But, how to decide which index partition is best for which document and query group? Let’s dive into query semantics.
What are query semantics?
Lexical semantics and query semantics are different from each other. “Buy” is an antonym of “Sell”, but for query semantics, they are “synonyms”. It means that the overall relevance of a “Buy” related document is equal to the overall relevance of a “Sell” document. Query semantics focus on the need behind the query. If the user’s general state is the same as another user’s from the same query group, they are in the same Markovian state. Sometimes, this is called “state bias”. In other words, a user always has a bias that comes from a state, and many user states follow each other with similar words, verbs, and nouns. Putting these concepts into the synonym format helps a search engine decrease computation needs while increasing the overall relevance and conceptual matching between user needs and the query.
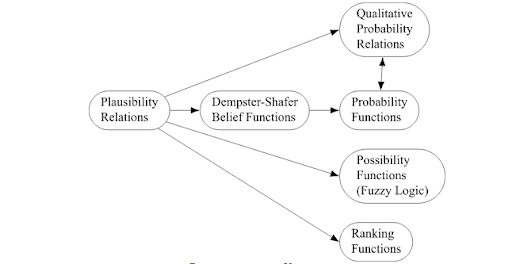
A schema of the Uncertain Inference from Stanford University
An example of index partition and quality thresholds
The query will be in “Verb + Noun” format. There are two candidate index partitions and the overall relevance will be calculated with basic IR (Information Retrieval) principles.
The web page uses the title “Best Verb + Methods for Small + Nouns”.
- The “noun” is plural.
- The word “method” qualifies as the “verb”.
- The “small” narrows the plural “noun” sets.
- The brand is not included.
- There are multiple methodologies for the “verb”.
- The person seeks “verb” related instructions and suggestions.
- The synonyms for the “method” are “approach”, “procedure”, “plans”, and “system”.
- The “smaller”, “tiny”, and “minor” are some possible synonyms.
- The word theme, and context shift from one synonym to another.
Since we only focus on basic IR principles, I won’t focus on web page layout, content format, language, or sentence structures.
Let’s create our index partitions based on the document clusters first.
- The first document cluster includes documents that contain the word “Best”, but not the “method”, it is for “Best + Nouns for People with Small X”.
- The second document cluster includes documents that contain the word “Best”, but not the small one, it is for “Best + Noun + Procedures for + Verb”.
- The third document cluster contains the correct verb and the noun, but it does not contain the best. It is for “Nouns + Verb for Small X”.
From a search engine’s point of view, the document doesn’t belong to any of these clusters absolutely. But, when we focus on query semantics, the search engine and the website operators will work together.
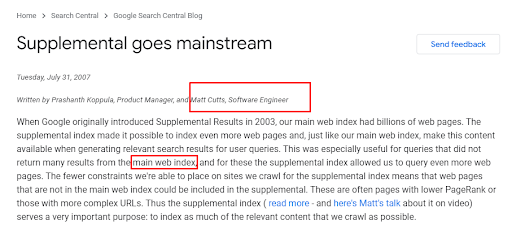
An explanation from Google, for Supplemental Index
An explanation from Google, for Supplemental Index.
If queries contain the word “method”, or “method” like words, it will have a heavier weight on the documents for IR score, even if there is no index partition for it. And, here, we start to get the “Continuous Information Retrieval” and “Automated Re-ranking” concepts. A search engine always creates new partitions of the index by taking the IR scores always-on, while re-ranking the documents.
If the queries contain the “method” more contextually, the specific query group will change the document clusters further. And, other documents from the supplemental index will be moved into the “main index”.
Thus, in topical authority and semantic SEO projects, the search engines start to change their document clusters based on the authoritative sources and their similarity to the others.
Most of the broad core algorithm updates focus on “relevance”, rather than the technical aspects of the web. They change the relevance of distance, semantic closeness, and co-occurrence matrices.
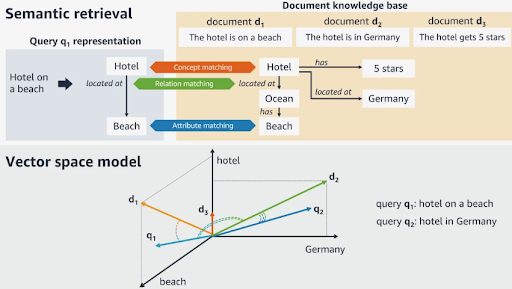
An example of Semantic Retrieval with vector search from Alessandro Moschitti
If there are enough levels of similar documents on the document cluster, the quality threshold will be higher. If not, the quality threshold will be smaller for relevance, and the search engines will choose to rank high PageRank sources even if their web pages are not a perfect match for the specific query. But they are relevant to the overall topic. From this last sentence, you can see the connection to passage indexing even further.
Since, in this example, we focused on only the index partition with IR, we didn’t mention the entities, attributes, or corroboration of web answers.
Why is topical authority relevant to quality thresholds?
Categorical quality is a subsection of topical authority that comes from Trystan Upstill. Categorical quality involves the source quality for certain types of queries. If a source contains the relevant n-grams, phrases, or phrase combinations for a source, it is given a higher category quality. The query categories are created based on the three things:
- Query phrase taxonomies
- Query taxonomy for search behavior
- Query phrase relevance
Categorical quality is measured with query processing and parsing output. A search engine can give different categorical quality scores to the different sources based on their overall relevance to certain query types.
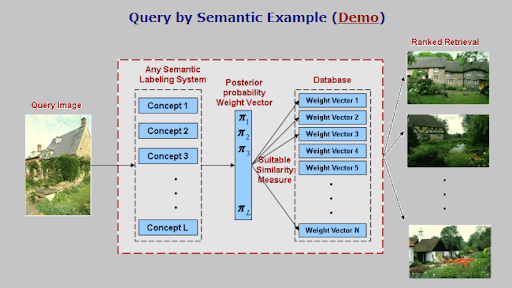
An example of Image Labeling and retrieval with visual queries
From our example IR score and index partition sample, you can understand that the overall categorical quality changes based on the overall relevance of the source to the overall query network. Thus, a topic consists of different sub-topics. The query networks are created based on topics and contexts. Thus, to have a topical authority for a topic, a brand, or source has to follow certain query networks.
And, different forms of verbs, nouns, adjectives, or query groups can cause changes in categorical quality while requiring changes in the semantic content network design. To exceed the quality threshold with the topical authority, the source has to prove that it has enough level of consolidation for depth, vastness, and publication activity with a proper document-query pair design.
What is the relevance of E-A-T to the quality thresholds?
E-A-T is a fundamental source filtration and prioritization concept for a group of signals that demonstrate the source’s overall quality and responsiveness to the user’s needs in a healthy way. E-A-T is related to the quality thresholds because even if a source has a topical authority, categorical quality, and overall relevance for certain query groups, if the source is not reliable, or trustworthy, the search engine can filter out the website.
Topical authority is one of the E-A-T signals, but they are not the same thing. A source with authority on a topic has to prove its expertise, information, and unique experience. These are E-A-T signals. But, E-A-T is a broader concept than topical authority. And, to exceed the quality threshold, the source shouldn’t have an E-A-T problem, or even if the source could rank initially, the re-ranking process would end with de-indexation.
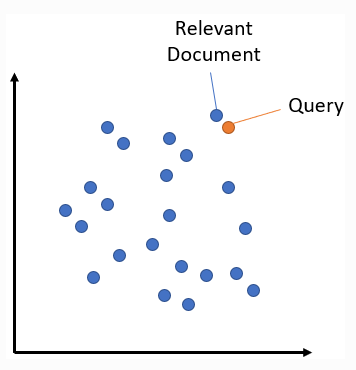
The Vector Search is relevant to quality thresholds. The vectors from queries and documents can be taken into similarity clusters to generate a relevance score.
How to understand the ranking algorithms for quality thresholds?
To understand the ranking algorithms of Google for quality thresholds, you have to understand initial ranking. The initial ranking of a web page is affected by specific relevance algorithms and information extraction processes. The overall quality of the source, its topical authority, PageRank, historical data, and E-A-T affect the initial ranking. The initial ranking score affects the overall re-ranking process and the historical data for the specific PageRank algorithms. In some Google designs and patents, the “first linked time” after the initial ranking shows the overall prominence and authority of the source and the quality of the web page. Because PageRank is an approver of the “predictive ranking”.
The quality thresholds are connected to the initial ranking rather than the re-ranking because the quality thresholds are meant to take the most quality sources and web pages into the main index by filtering them. The initial ranking success of a web page from a source affects the other web page’s possibility to rank for the same categorical query groups. Because predictive ranking and information retrieval focus on saving time and energy from the ranking by trusting certain sources.
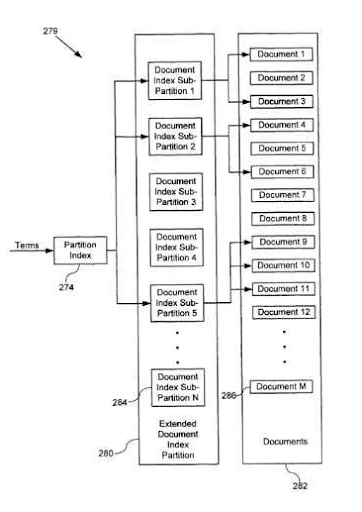
An example of Index Partitioning with Supplemental and Main Index.
An example of Index Partitioning with Supplemental and Main Index.
To improve a source for the overall ranking, and exceed the quality thresholds, the source has to create a difference from the previous state, whether by publishing new content or updating the existing content.
To understand Google’s ranking algorithms, read the Initial and Re-ranking Case Study.
How to determine quality thresholds?
Quality thresholds come from the cornerstone sources. In other words, the most qualified source from the SERP and the least quality source from the SERP create the quality range of the documents. These sources and their web pages create the web page and source types to be ranked. A search engine can promote certain types of sources on the SERP if algorithms think that they are a better fit for user behaviors. For a single-word query, it is hard to decide what is the user behavior or user purpose after the search. Thus, the search engine has to use information foraging to construct a multi-layered and diverse SERP with different source types.
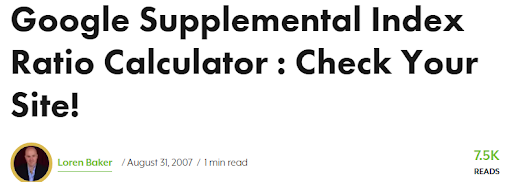
There were even tools to check the ratio of Supplementally Indexed document ratio for Google SEO.
For every source type, there is a different quality threshold. A website from the second SERP web page can’t focus on all its first web page competitors because search engines compare websites to other websites according to their type, particularly if a query result contains the following website types:
3 E-commerce websites
2 informational websites
4 general bloggers
1 video website result
To determine the quality thresholds for a SERP instance, the website’s type, identity, and context have to be determined because based on the comparative ranking, the source will be compared to different types. A search engine compares website types to website types first. In other words, in broad core algorithm updates, it is not simply websites competing against a website. An entire cluster of websites competes against other clusters of websites. And, every cluster has a different representative sample.
In the video below, I explain the topical authority and representative source concepts further.
Updates of the Quality Threshold SEO Case Study
The updates of the Quality Threshold SEO Case Study involve the changes that occurred the two weeks following the initial publication on Oncrawl.
After publishing the Quality Threshold SEO Case Study, Google’s ongoing updates, and historical data accumulation guaranteed the positive ranking state with regular organic search performance increases.
Including the last two weeks, you can see the evident increase that is stated and the foreshadowed organic traffic increase via Quality Threshold Exceed.
The last six months of comparison of the SEO Case Study’s subject websites Google searches are below.
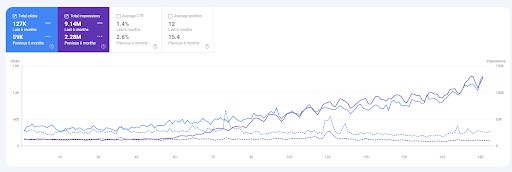
115.25% organic click increase, 308.87% organic impression increase, 21.4% average position increase.
The last three months of comparison:
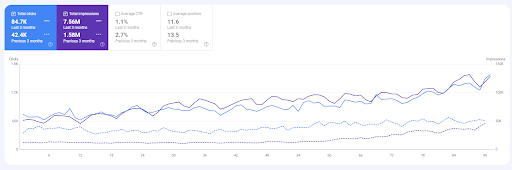
99,45% Organic Click Increase, 378.48% Organic Impression Increase, 16.37% Organic Search Results Position Increase.
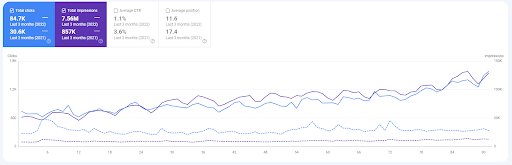
176.79% Organic Click Increase, 789.41% Organic Impression Increase, %34.09 Organic Search Results Position Increase.
Below, you can see the version of the Ahrefs organic search result and performance data for the Importance of Quality Thresholds for SEO Case Study’s subject website from 16 days later.
There are 6,000 more queries with 27,000 organic traffic sessions calculated by Ahrefs.
The version of the SEMrush organic search performance data for the same website from 16 days later shows 34,000 new organic clicks and nearly 10,000 new organic queries. To demonstrate that the source dominates against the health niche authorities with only 27 well-written articles, you can check the sections below.
There are 13,000 common queries with Webmd.com. And, for nearly 3,000 of these, the subject website dominates Webmd.com. Additionally, the average keyword difficulty is over 85.
There are more than 11,000 common queries against Healthline.com. For more than 2,800 of them, the average keyword of the Subject Website dominates Healthline.com.
There are more global authority websites on health and YMYL topics and lose against the subject website. The queries above are random samples that are chosen against random sources. Below, you can see the latest re-ranking direction and magnitude for the subject website.
Last 16 months of GSC data of the subject website. It shows a consistent flat line until publishing the last 27 articles that were written algorithmically. To understand the algorithmic authorship further, read Semantic Networks for SEO.
To demonstrate the authority of the content and authenticity of the author (main content creator), you should understand the Knowledge Domain Expert. A knowledge domain expert is a primary source of information for a topic, and different contexts in the topical nets. Proving authenticity and authority of the information to a semantic search engine changes the identity of a source (website).
Why does a search engine need a quality threshold?
Quality thresholds are used in nearly all ranking, filtering, and sorting algorithms based on certain metrics.
For example, to classify an 800×600 image, a machine learning algorithm needs 58 million different “bundle boxes”, but it is not possible to do it because it is costly. Thus, search engines use “Region Proposals” with “Selective Search” for object detection. And, if there are 5 different objects, it will be hard to label the image. Thus, a threshold is a must.
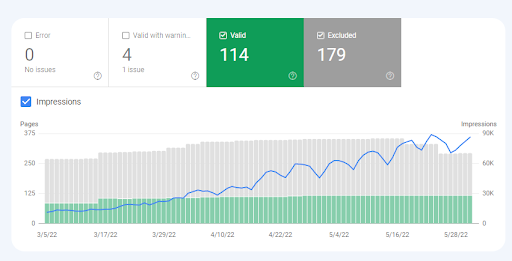
This is a small website
Another example of quality thresholds are supplemental indexes. The Charles Darwin of SEO, Bill Slawski, was the first person who mentioned the concept of the supplemental index by Google. According to design, Google uses a supplemental index and the main index. If a source exceeds the quality threshold, it changes its position from the supplemental index to the main index.
Let’s look at another example of the quality threshold that happens with indexation. Google uses predictive quality understanding by checking the initial URLs from the crawl queue to see whether it’s worth a deeper crawl or not.
A final example of the quality threshold is from News SEO; if Google doesn’t see a proper summary at the introduction of the article, they can skip the article for further ranking purposes. Thus, the quality of the summary can affect the overall ranking, even if it is not the quality of the entire article.
Note: The actual version of the SEO Case Study will be given during the Semantic SEO Course with 5 more websites, actual research papers, and more information.
Some other sample websites and industries for initial ranking with exceeded quality thresholds
Before moving further, you can see some other website samples that exceed the quality thresholds from different industries.
The sample below is an e-commerce store for certain gift products, and there is a planned launch. Thus, it has been indexed and served faster.
The example below is again from a YMYL website with a quick launch for exceeding quality thresholds.
The sample below is from a health industry website. You can see that “predictive ranking” works with different confidence levels.
The sample below is another quality threshold exceeded, and it is a local search directory for offline businesses for commercial activities. Its main benefit is stocking information.
The last sample below is an exact match domain for YMYL Industry, used cars.
These samples will be given in the Semantic SEO Course with more detailed scientific explanations. All these launches focused on specific types of query networks with an understanding of the ranking algorithms of Google, and quality thresholds with predictive ranking. Since all the samples are YMYL websites and touch the health, it is more crucial to state that it is possible to take traffic from global authorities without waiting that long.
Why is index partition necessary for a search engine?
Index partition means that a search engine divides its index into multiple parts. When a user enters a query to a search engine, do you think that a search engine checks the entire index? Then, you would wait two hours for a single SERP. And, what if a search engine searches the possible queries from the users even before the user searches for it to create document clusters?
This is called synthetic queries, which I explained in my Semantic Search Engine and Query Processing methodology. The search engine’s purpose with index partition is to decide which document and query will go to which cluster of concepts. And, there are different index partition methods. The latest methods focus on Triples (Subject, Predicate, Object). Previous samples focused on the context terms and head words in the documents.
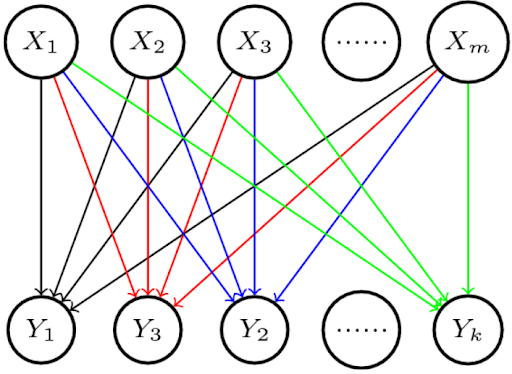
An example of Uncertain Inference for queries
Index partition and quality thresholds are related to each other.
How to decide which document goes to which cluster?
A search engine can index a document, then de-index and re-index it for another query. A query in the form of a verb can have different lemmatizations, and the search engine can create different index partitions for different verb formats. The document might not have the specific verb formats, or the specific verb + noun sequences, or even if it does, it might give incorrect information which contains the topic of a knowledge base. Thus, different quality thresholds are determined for different formats of the nouns, verbs, and other types of words such as adjectives.
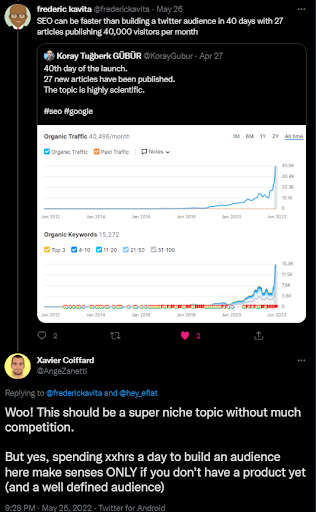
I didn’t write this case study against these claims. But I want to change how SEO is being done.
Relevance works to decide the overall connectedness between a document and the query. If the document has multiple macro and micro-contexts, it might be a contextless, or wrongly formatted document. For these types of situations, the search engine has passage indexing, which is a method to eliminate most of the irrelevant sections of the document and rank it with only that specific passage.
But, how to decide which index partition is best for which document and query group? Let’s dive into query semantics.
What are query semantics?
Lexical semantics and query semantics are different from each other. “Buy” is an antonym of “Sell”, but for query semantics, they are “synonyms”. It means that the overall relevance of a “Buy” related document is equal to the overall relevance of a “Sell” document. Query semantics focus on the need behind the query. If the user’s general state is the same as another user’s from the same query group, they are in the same Markovian state. Sometimes, this is called “state bias”.
In other words, a user always has a bias that comes from a state, and many user states follow each other with similar words, verbs, and nouns. Putting these concepts into the synonym format helps a search engine decrease computation needs while increasing the overall relevance and conceptual matching between user needs and the query.
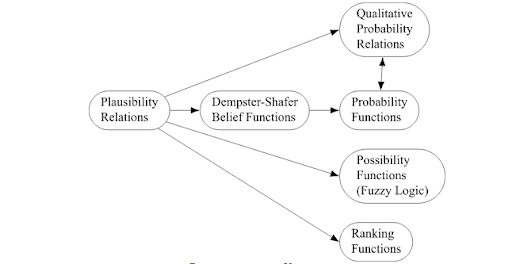
A schema of the Uncertain Inference from Stanford University
An example of index partition and quality thresholds
The query will be in “Verb + Noun” format. There are two candidate index partitions and the overall relevance will be calculated with basic IR (Information Retrieval) principles.
The web page uses the title “Best Verb + Methods for Small + Nouns”.
- The “noun” is plural.
- The word “method” qualifies as the “verb”.
- The “small” narrows the plural “noun” sets.
- The brand is not included.
- There are multiple methodologies for the “verb”.
- The person seeks “verb” related instructions and suggestions.
- The synonyms for the “method” are “approach”, “procedure”, “plans”, and “system”.
- The “smaller”, “tiny”, and “minor” are some possible synonyms.
- The word theme, and context shift from one synonym to another.
Since we only focus on basic IR principles, I won’t focus on web page layout, content format, language, or sentence structures.
Let’s create our index partitions based on the document clusters first.
- The first document cluster includes documents that contain the word “Best”, but not the “method”, it is for “Best + Nouns for People with Small X”.
- The second document cluster includes documents that contain the word “Best”, but not the small one, it is for “Best + Noun + Procedures for + Verb”.
- The third document cluster contains the correct verb and the noun, but it does not contain the best. It is for “Nouns + Verb for Small X”.
From a search engine’s point of view, the document doesn’t belong to any of these clusters absolutely. But, when we focus on query semantics, the search engine and the website operators will work together.
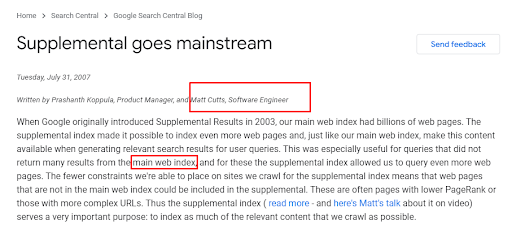
An explanation from Google, for Supplemental Index
If queries contain the word “method”, or “method” like words, it will have a heavier weight on the documents for IR score, even if there is no index partition for it. And, here, we start to get the “Continuous Information Retrieval” and “Automated Re-ranking” concepts. A search engine always creates new partitions of the index by taking the IR scores always-on, while re-ranking the documents.
If the queries contain the “method” more contextually, the specific query group will change the document clusters further. And, other documents from the supplemental index will be moved into the “main index”.
Thus, in topical authority and semantic SEO projects, the search engines start to change their document clusters based on the authoritative sources and their similarity to the others.
Most of the broad core algorithm updates focus on “relevance”, rather than the technical aspects of the web. They change the relevance of distance, semantic closeness, and co-occurrence matrices.
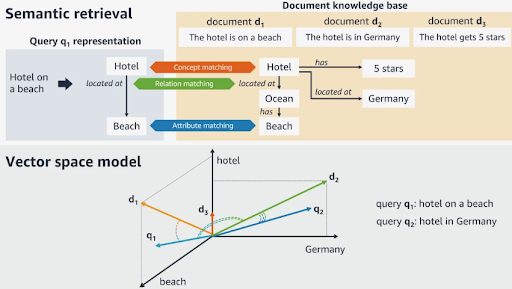
An example of Semantic Retrieval with vector search from Alessandro Moschitti
If there are enough levels of similar documents on the document cluster, the quality threshold will be higher. If not, the quality threshold will be smaller for relevance, and the search engines will choose to rank high PageRank sources even if their web pages are not a perfect match for the specific query. But they are relevant to the overall topic. From this last sentence, you can see the connection to passage indexing even further.
Since, in this example, we focused on only the index partition with IR, we didn’t mention the entities, attributes, or corroboration of web answers.
Why is topical authority relevant to quality thresholds?
Categorical quality is a subsection of topical authority that comes from Trystan Upstill. Categorical quality involves the source quality for certain types of queries. If a source contains the relevant n-grams, phrases, or phrase combinations for a source, it is given a higher category quality. The query categories are created based on the three things:
- Query phrase taxonomies
- Query taxonomy for search behavior
- Query phrase relevance
Categorical quality is measured with query processing and parsing output. A search engine can give different categorical quality scores to the different sources based on their overall relevance to certain query types.
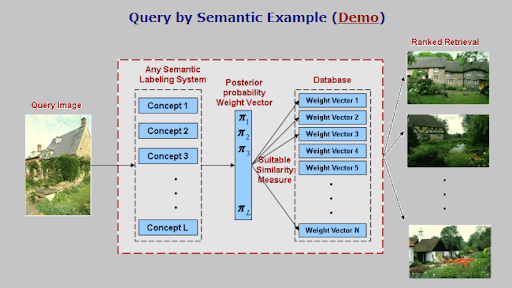
An example of Image Labeling and retrieval with visual queries
From our example IR score and index partition sample, you can understand that the overall categorical quality changes based on the overall relevance of the source to the overall query network. Thus, a topic consists of different sub-topics. The query networks are created based on topics and contexts. Thus, to have a topical authority for a topic, a brand, or source has to follow certain query networks.
And, different forms of verbs, nouns, adjectives, or query groups can cause changes in categorical quality while requiring changes in the semantic content network design. To exceed the quality threshold with the topical authority, the source has to prove that it has enough level of consolidation for depth, vastness, and publication activity with a proper document-query pair design.
What is the relevance of E-A-T to the quality thresholds?
E-A-T is a fundamental source filtration and prioritization concept for a group of signals that demonstrate the source’s overall quality and responsiveness to the user’s needs in a healthy way. E-A-T is related to quality thresholds because even if a source has topical authority, categorical quality, and overall relevance for certain query groups, if the source is not reliable, or trustworthy, the search engine can filter out the website.
Topical authority is one of the E-A-T signals, but they are not the same thing. A source with authority on a topic has to prove its expertise, information, and unique experience. These are E-A-T signals. But, E-A-T is a broader concept than topical authority. And, to exceed the quality threshold, the source shouldn’t have an E-A-T problem, or even if the source could rank initially, the re-ranking process would end with de-indexation.
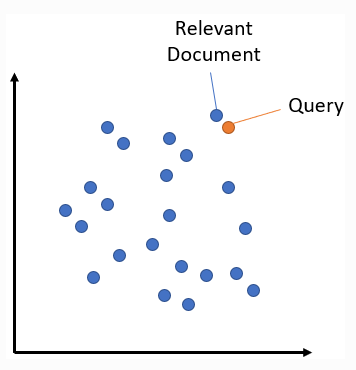
The Vector Search is relevant to quality thresholds. The vectors from queries and documents can be taken into similarity clusters to generate a relevance score
How to understand the ranking algorithms for quality thresholds?
To understand the ranking algorithms of Google for quality thresholds, you have to understand initial ranking. The initial ranking of a web page is affected by specific relevance algorithms and information extraction processes. The overall quality of the source, its topical authority, PageRank, historical data, and E-A-T affect the initial ranking.
The initial ranking score affects the overall re-ranking process and the historical data for the specific PageRank algorithms. In some Google designs and patents, the “first linked time” after the initial ranking shows the overall prominence and authority of the source and the quality of the web page. Because PageRank is an approver of the “predictive ranking”.
The quality thresholds are connected to the initial ranking rather than the re-ranking because the quality thresholds are meant to take the highest quality sources and web pages into the main index by filtering them. The initial ranking success of a web page from a source affects the other web page’s possibility to rank for the same categorical query groups. Because predictive ranking and information retrieval focus on saving time and energy from the ranking by trusting certain sources.
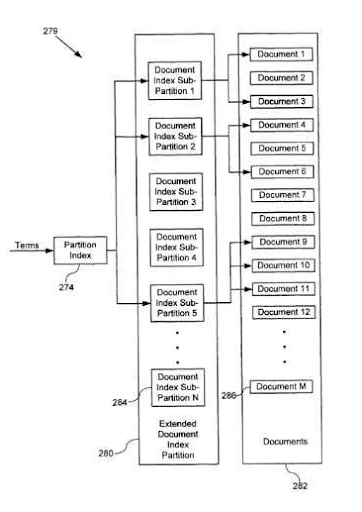
An example of Index Partitioning with Supplemental and Main Index
To improve a source for the overall ranking, and exceed the quality thresholds, the source has to create a difference from the previous state, whether by publishing new content or updating the existing content.
To understand Google’s ranking algorithms, read the Initial and Re-ranking Case Study.
How to determine quality thresholds?
Quality thresholds come from the cornerstone sources. In other words, the most qualified source from the SERP and the least quality source from the SERP create the quality range of the documents. These sources and their web pages create the web page and source types to be ranked. A search engine can promote certain types of sources on the SERP if algorithms think that they are a better fit for user behaviors. For a single-word query, it is hard to decide what is the user behavior or user purpose after the search. Thus, the search engine has to use information foraging to construct a multi-layered and diverse SERP with different source types.
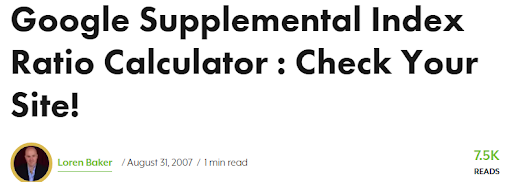
There were even tools to check the ratio of Supplementally Indexed document ratio for Google SEO.
For every source type, there is a different quality threshold. A website from the second SERP web page can’t focus on all its first web page competitors because search engines compare websites to other websites according to their type, particularly if a query result contains the following website types:
- E-commerce websites
- Informational websites
- General bloggers
- Video website result
To determine the quality thresholds for a SERP instance, the website’s type, identity, and context have to be determined because based on the comparative ranking, the source will be compared to different types. A search engine compares website types to website types first. In other words, in broad core algorithm updates, it is not simply websites competing against a website. An entire cluster of websites competes against other clusters of websites. And, every cluster has a different representative sample.
In the video below, I explain the topical authority and representative source concepts further.
To determine the quality thresholds for a website, SEO has to read the mind of the search engine. From qualitative to quantitative, there should be different metrics to consider and optimize, such as n-gram count, skip-gram dominant words, site-wide n-grams, sentence structures, nouns and predicates, stylometry of the documents, percentages, numeric values, certain types of entities’ dominance, the accuracy of the information, image count, needs, and image objects, image labels, video need, the identity of the owner of the company and more.
According to the results, and possibilities of the project, SEO should create a different balance between quality and quantity for different metrics to exceed the quality thresholds quickly to be able to take traffic from the websites.
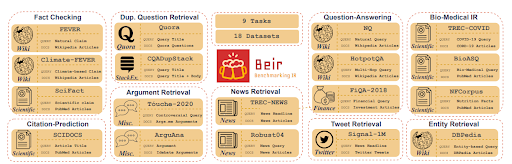
An example of benchmarking of information retrieval models with different tasks. All these belong to specific semantic search engine language model tasks.
How to make a search engine reorganize index partitions?
To make a search engine reorganize the index partitions, the central concepts and seed queries should be used with different information and co-occurrence matrices. A new information graph and semantic content network can force Google to reorganize context domains and knowledge domains. The way it works is that Google chooses a representative source for a topic and ranks other sources accordingly, if they are similar or qualify for the same level of signals as the representative source.
I call this concept “source shadowing”; the idea that one website can appropriate the rankings of another site by shadowing it and consequently become the representative site. Additionally, in regards to source shadowing – or forcing a search engine to reorganize queries and document clusters – the source has to exceed the quality threshold for a topic and change the conceptual connections and entity-attribute relations with a high level of confidence. In other words, it is about the “corroboration of web answers” and choosing a “truth authority”.
If one source creates a better information tree, search engines will understand the same topic easier and with higher confidence. Additionally, since the bottom and upper-quality thresholds are exceeded by a new information source that doesn’t have an E-A-T problem, the website can outrank all the specific high-quality global authority websites. Publication frequency and convincing comprehensiveness-related algorithms across different web pages with consistent declarations and truthful information are important factors that help search engines avoid the “embarrassment factor”.
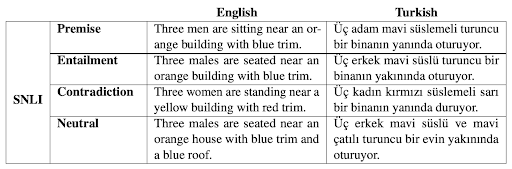
NLI, Natural Language Inference, should be understood for creating quality documents
How to exceed quality thresholds?
To exceed quality thresholds, implement the methodologies below.
- Use factual sentence structures. (X is known for Y → X does Y)
- Use research and university studies to prove the point. (X provides Y → According to X University research from Z Department, on C Date, the P provides Y)
- Be concise, do not use fluff in the sentence. (X is the most common Y → X is Y with D%)
- Include branded images with a unique composition.
- Have all the EXIF data and licenses of the images.
- Do not break the context across different paragraphs.
- Optimize discourse integration.
- Opt for the question and answer format.
- Do not distance the question from the answer.
- Expand the evidence with variations.
- Do not break the information graph with complicated transitions. Follow a logical order in the declarations.
- Use shorter sentences as much as possible.
- Decrease the count of contextless words in the document.
- If a word doesn’t change the meaning of the sentence, delete it.
- Put distance between the anchor texts and the internal links.
- Give more information per web page, per section, per paragraph, and sentence.
- Create a proper context vector from H1 to the last heading of the document.
- Focus on query semantics with uncertain inference.
- Always give consistent declarations and do not change opinion, or statements, from web page to web page.
- Use a consistent style across the documents to show the brand identity of the document.
- Do not have any E-A-T negativity, have news about the brand with a physical address and facility.
- Be reviewed by the competitors or industry enthusiasts.
- Increase the “relative quality difference” between the source’s current and previous state to trigger a re-ranking.
- Include the highest quality documents as links on the homepage so they are visible to crawlers as early as earlier.
- Have unique n-grams, and phrase combinations to show the originality of the document.
- Include multiple examples, data points, data sets, and percentages for each information point.
- Include more entities, and attribute values, as long as they are relevant to the macro context.
- Include a single macro context for every web page.
- Complete a single topic with every detail, even if it doesn’t appear in the queries, and the competitor documents.
- Reformat the document in an infographic, audio, or video to create a better web surface coverage.
- Use the internal links to cover the same seed query’s subqueries with variations of the phrase taxonomies.
- Use fewer links per document while adding more information.
- Use the FAQ and article structured data to consistently communicate with the search engine from every level and for every data extraction algorithm.
- Have a concrete authorship authority for your own topic.
- Do not promote the products while giving information about health.
- Use branded CTAs by differentiating them from the main context and structure of the document.
- Refresh at least 30% of the source by increasing the relative difference. (Main matter is explained in the full version of the SEO Case Study.)
What is predictive ranking?
Predictive ranking relies on predictive information retrieval in a cost-effective way. Predictive ranking predicts an optimum ranking capacity and spot for the source and the web pages of the source with a certain confidence. If the rankings are volatile for certain queries, it means that the search engine is not confident about the ranking, and tries to predict new values.
Regarding predictive ranking or overall ranking algorithms, there is no certainty. Thus, search engines shouldn’t be evaluated in a deterministic way. It should be evaluated with a probabilistic understanding. The predictive ranking algorithms might use the implicit user feedback, the information gap, and information content of the documents along with the PageRank, and reference intensity of the sources for certain topics. For quality thresholds, predictive ranking provides a dynamic change in the information retrieval score threshold while dividing the indexes into further sub-parts and sections.
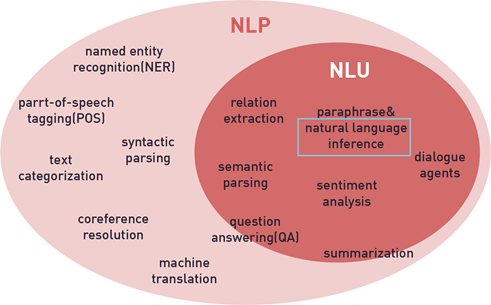
Different NLP tasks and understanding are prominent to see semantic search engine’s priorities.
Why are predictive ranking and uncertain inference prominent?
Uncertain inference is a concept of information retrieval that was first described by C.J. van Rijsbergen, a concept leader for search engines from Glasgow University and the founder of the IR Club.
Uncertain inference doesn’t focus on a single query, but instead focuses on all the connected queries, phrase variations, and synonyms related to the initial query. However, that is not all the concept covers; it also mentions that a “knowledge base”, a repository of facts, is necessary to infer the meaning of a query in a factual way.
The query path, or the sequential queries, are necessary to understand the user’s state of mind and the connections between the concepts. Predictive ranking is relevant to uncertain inference because it is not easy for a search engine to see the connections. If a search engine can’t see the connections between things, there are two possibilities:
- Classical IR
- Classical PageRank
These two methods worked great for 20 years, but, now, even a small search engine brand can create a factual repository via open-source common databases and rank documents better than Google. Another disadvantage is that new search engines do not have a problem with further crawling, or storage, and they do not need that much data for ranking documents with information understanding.
It means Google has to push harder in three areas:
- Ambiance Optimization
- Information Accuracy
- Information Connections
The first one is another topic, but the last two are directly related to semantic SEO and linguistics. If a search engine understands the state of the users better, the content is suggested to users without the user knowing or searching for the specific concept. Thus, a proper information structure and graph, and a better connection between things from the documents, help a search engine to infer the physical world. It’s important to understand that search engines index the physical world via the digital world. This is the main summary of entity-oriented search.
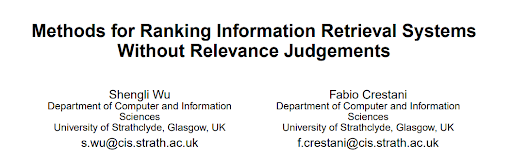
There is different research to compare IR Models to each other. One of them focuses on reference count from the IR Models for certain documents. If different IR Models reference the same document, it means that the document is relevant for all possibilities.
Last thoughts on the Quality Threshold SEO Case Study
Quality thresholds exist in every query network to provide qualitative and quantitative ranking filtration. These thresholds can differ from topic to topic or representative source to another representative source. Quality thresholds should be seen as the main ranking threshold for sources. And, every SEO should calculate the thresholds to dominate their own niche with a better structured, disciplined mindset for SEO.
I started this SEO Case Study with the Charles Darwin of SEO, Bill Slawski. Most of these concepts and theories came from his theoretical search philosophy. Let’s remember the name Bill Slawski, always.